I work on FrankenPHP quite a bit, which is a PHP SAPI written in Go. For the uninitiated, PHP is written in C, so FrankenPHP uses cgo to interface with PHP. This also means that it uses Handles.
Just to give you a bit of a background on Handles and what they are used for: in cgo, you can’t pass pointers back and forth between C and Go. This is because the memory locations are likely to change during runtime. So, Go provides “Handles” that are a map between a number and a Go interface. Thus, if you want to pass something to C, then when it gets passed back to Go, we can look up that handle using the number and get the Go value.
In essence, this works “good enough” for most applications. The only issue is that it uses a sync.Map
under the hood. This means that if we receive 1,000 requests at exactly the same time, we can only process them one at a time. Granted, the current implementation of sync.Map
is quite fast… so, can we improve it?
First, let’s get an idea of how fast it currently is by performing a benchmark. There is only one problem with Go’s current benchmarks: they don’t really show concurrent performance.
Let me illustrate. Here’s the “default” output of the current Handle benchmarks:
BenchmarkHandle BenchmarkHandle/non-concurrent BenchmarkHandle/non-concurrent-16 2665556 448.9 ns/op BenchmarkHandle/concurrent BenchmarkHandle/concurrent-16 2076867 562.3 ns/op
This doesn’t show us anything about the scale of the problem nor what we are solving for. So, I’m going to update the benchmark code a bit to show what is happening:
func formatNum(n int) string { // add commas to separate thousands s := fmt.Sprint(n) if n < 0 { return "-" + formatNum(-n) } if len(s) <= 3 { return s } return formatNum(n/1000) + "," + s[len(s)-3:] } func BenchmarkHandle(b *testing.B) { b.Run("non-concurrent", func(b *testing.B) { for i := 0; i < b.N; i++ { h := NewHandle(i) _ = h.Value() h.Delete() } }) b.Run("concurrent", func(b *testing.B) { start := time.Now() b.RunParallel(func(pb *testing.PB) { var v int for pb.Next() { h := NewHandle(v) _ = h.Value() h.Delete() } }) fmt.Printf("Time: %fs\tTime Each: %dns\tNumber of handles: %s\n", time.Since(start).Seconds(), time.Since(start).Nanoseconds()/int64(b.N), formatNum(b.N)) }) }
Now, let us see the output!
goos: linux goarch: amd64 pkg: runtime/cgo cpu: 11th Gen Intel(R) Core(TM) i7-11800H @ 2.30GHz BenchmarkHandle BenchmarkHandle/non-concurrent BenchmarkHandle/non-concurrent-16 2618832 459.4 ns/op BenchmarkHandle/concurrent Time: 0.000045s Time Each: 44873ns Number of handles: 1 Time: 0.000063s Time Each: 634ns Number of handles: 100 Time: 0.006349s Time Each: 634ns Number of handles: 10,000 Time: 0.561490s Time Each: 561ns Number of handles: 1,000,000 Time: 1.210851s Time Each: 566ns Number of handles: 2,137,053 BenchmarkHandle/concurrent-16 2137053 566.6 ns/op
Here we can see what is going on under the hood. The benchmark tool actually runs several benchmarks increasing the number of iterations and estimates how many it can do in about 1 second.
Now, we can kind of see our problem: we hit a ceiling on how fast we can create handles. Getting 100 thousand requests at once (not uncommon in a load test) means a minimum latency of ~6 milliseconds for the last request.
How could we implement this in a lock-free way, without using mutexes (or at least, keeping them to a minimum)?
I went for a walk and thought about it. The best I could come up with was some clever usages of slices and partitions. Then I implemented it. (you can see that implementation here, but I’m not going to talk about it)
Once I submitted that patch to Go, it was immediately “held” since it introduced a new API. Thus, I went back to the drawing board to figure out how to implement it without changing the API.
Essentially, it boils down to thinking about how we can cleverly use slices and trade some memory for speed. I started with a basic slice of atomic pointers ([]*atomic.Pointer[slot]
). Now, for those who don’t know about Atomics in Go, we have to use the same type of all things in the atomic. An Atomic can only hold nil
initially, after that, it must hold the same value. So, I needed to create a new type to hold the values: “slots.”
// slot represents a slot in the handles slice for concurrent access. type slot struct { value any }
But we still need some way to indicate that the value is empty, and it needs to be fast. Thus we will create a “nil slot” that can be easily checked for equality (we can just look at the address).
And finally, we need to atomically be able to grow the slice of slots and keep track of the size atomically as well. Altogether, this results in the following data structures:
// slot represents a slot in the handles slice for concurrent access. type slot struct { value any } var ( handles []*atomic.Pointer[slot] releasedIdx sync.Pool nilSlot = &slot{} growLock sync.RWMutex handLen atomic.Uint64 ) func init() { handles = make([]*atomic.Pointer[slot], 0) handLen = atomic.Uint64{} releasedIdx.New = func() interface{} { return nil } growLock = sync.RWMutex{} }
Oh, and we also need a “free-list” to keep track of the freed indices for reuse. We’ll use a sync.Pool
which keeps a thread-local list and falls back to a global one if necessary. It is very fast!
Creating a Handle
Now that we have all the parts necessary to store our handles, we can go about creating a new handle. Here’s the basic algorithm:
- Set the current handle integer to 1
- Create a
slot
for the data - for ever:
- Check the
sync.Pool
for a released handle- if there is one, set the current handle to it
- See if the current handle is larger than the slice length, if so, grow it
- Try a compare and swap for
nil
on the current handle- if it fails, increase the current handle by one
- if it succeeds, we have our handle
- goto 3
For the smart people out there, you may notice this is O(n)
in the worst case and should be “slower” than sync.Map
since, according to ChatGPT, it should be O(1)
to store in the map.
However, we are not trying to be “faster,” but lock-free.
The actual implementation looks like this:
func NewHandle(v any) Handle { var h uint64 = 1 s := &slot{value: v} for { if released := releasedIdx.Get(); released != nil { h = released.(uint64) } if h > handLen.Load() { growLock.Lock() handles = append(handles, &atomic.Pointer[slot]{}) handLen.Store(uint64(len(handles))) growLock.Unlock() } growLock.RLock() if handles[h-1].CompareAndSwap(nilSlot, s) { growLock.RUnlock() return Handle(h) } else if handles[h-1].CompareAndSwap(nil, s) { growLock.RUnlock() return Handle(h) } else { h++ } growLock.RUnlock() } }
Retrieving a value is O(1)
, however:
func (h Handle) Value() any { growLock.RLock() defer growLock.RUnlock() if h > Handle(len(handles)) { panic("runtime/cgo: misuse of an invalid Handle") } v := handles[h-1].Load() if v == nil || v == nilSlot { panic("runtime/cgo: misuse of an released Handle") } return v.value }
As is deleting:
func (h Handle) Delete() { growLock.RLock() defer growLock.RUnlock() if h == 0 { panic("runtime/cgo: misuse of an zero Handle") } if h > Handle(len(handles)) { panic("runtime/cgo: misuse of an invalid Handle") } if v := handles[h-1].Swap(nilSlot); v == nil || v == nilSlot { panic("runtime/cgo: misuse of an released Handle") } releasedIdx.Put(uint64(h)) }
When we put it all together, we can run our benchmarks:
goos: linux goarch: amd64 pkg: runtime/cgo cpu: 11th Gen Intel(R) Core(TM) i7-11800H @ 2.30GHz BenchmarkHandle BenchmarkHandle/non-concurrent BenchmarkHandle/non-concurrent-16 11961500 99.17 ns/op BenchmarkHandle/concurrent Time: 0.000016s Time Each: 15950ns Number of handles: 1 Time: 0.000027s Time Each: 272ns Number of handles: 100 Time: 0.001407s Time Each: 140ns Number of handles: 10,000 Time: 0.103819s Time Each: 103ns Number of handles: 1,000,000 Time: 1.129587s Time Each: 97ns Number of handles: 11,552,226 BenchmarkHandle/concurrent-16 11552226 97.78 ns/op
Now, even if we got one million requests at the same time, our latency would only be about 10 milliseconds. Previously, just from handles, our max requests-per-second were ~1.7 million. Now, it is ~10.2 million.
This is a huge speed-up for such a micro-optimization… but what is the actual performance gain in FrankenPHP and what is the “worst-case” of O(n)
actually look like?
goos: linux goarch: amd64 pkg: runtime/cgo cpu: 11th Gen Intel(R) Core(TM) i7-11800H @ 2.30GHz BenchmarkHandle BenchmarkHandle/non-concurrent BenchmarkHandle/non-concurrent-16 11620329 99.21 ns/op BenchmarkHandle/concurrent Time: 0.000020s Time Each: 20429ns Number of handles: 1 Time: 0.000019s Time Each: 193ns Number of handles: 100 Time: 0.002410s Time Each: 240ns Number of handles: 10,000 Time: 0.248238s Time Each: 248ns Number of handles: 1,000,000 Time: 1.216321s Time Each: 251ns Number of handles: 4,833,445 BenchmarkHandle/concurrent-16 4833445 251.7 ns/op
In the worst case (ignoring our “free-list”) we are still handily faster than the original implementation (about twice as fast).
For those of you who like graphs, here it is:
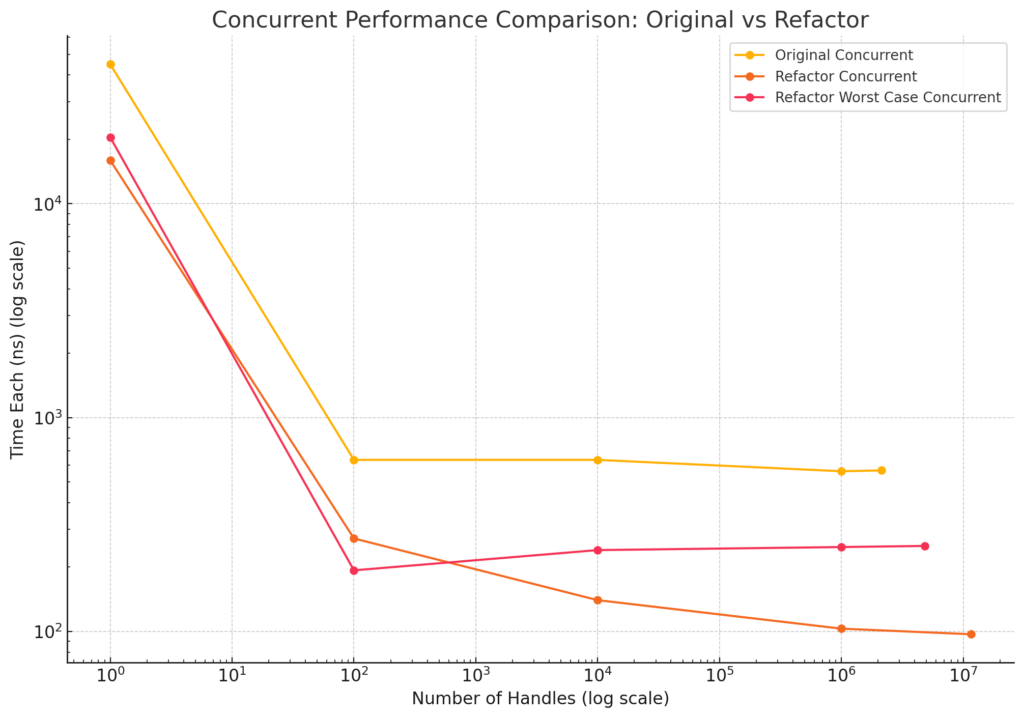
For FrankenPHP, it results in a 1-5% increase in requests-per-second. So, while this does measurably improve the performance of FrankenPHP, it doesn’t equate to the 5x improvement we’d expect; which means it is time to go hunting for more improvements in Go.
I’ve submitted a CL to Go with these improvements: https://go-review.googlesource.com/c/go/+/600875 but I have no idea if they will ever review it or consider it. From Go’s perspective (and most developers) this isn’t usually something on a hot-path that would benefit from such a micro-optimization. It’s only when handles are being created on a hot-path (such as in FrankenPHP’s case) that any performance gains can be realized.